Top 5 AI Trends to Watch in 2025 for Data Privacy
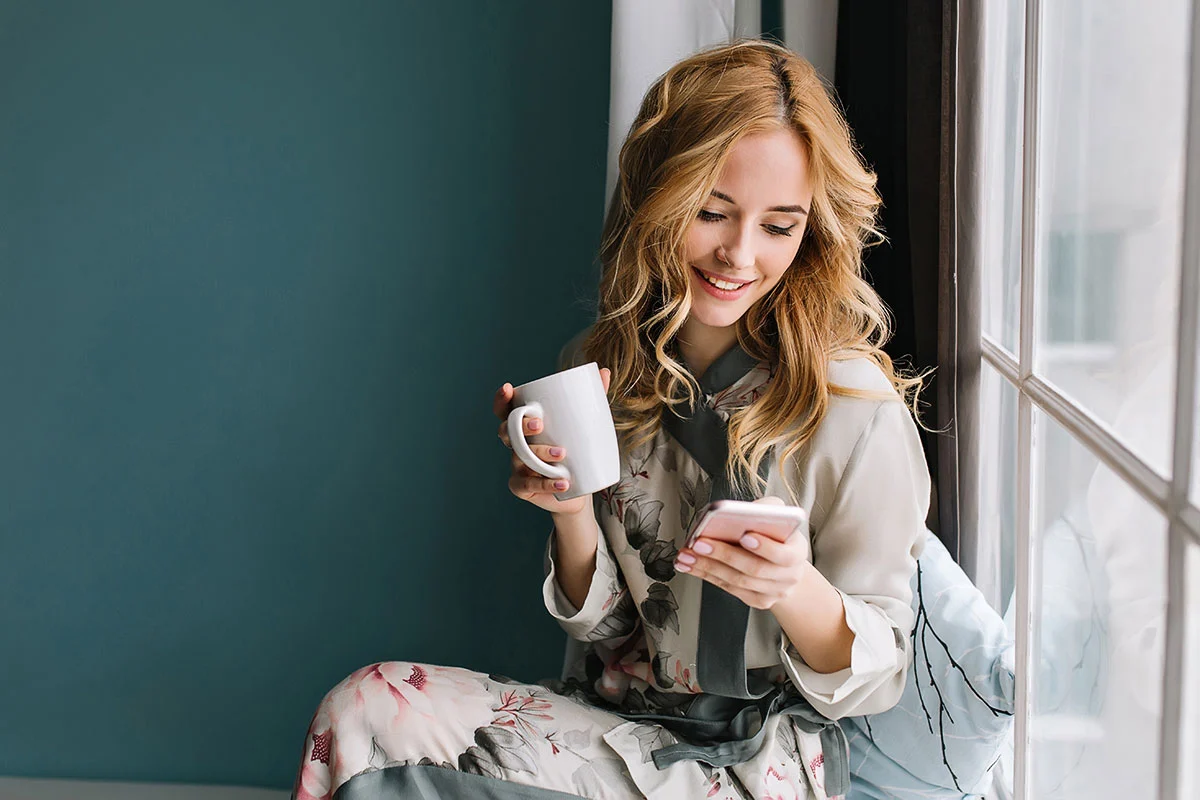
As artificial intelligence (AI) continues to revolutionize industries, its implications for data privacy have become a focal point. In 2025, emerging trends in AI will shape how organizations collect, process, and protect sensitive information. This article highlights the top five AI trends in data privacy and provides practical examples of their impact.
1. Privacy-Enhancing AI Technologies (PETs)
Privacy-enhancing technologies (PETs) are at the forefront of AI innovation, enabling organizations to analyze data while minimizing privacy risks. These technologies allow sensitive information to be used without exposing individual identities.
Key Examples:
- Federated Learning: AI models are trained on decentralized data stored on user devices instead of transferring it to central servers. For instance, Google’s Gboard uses federated learning to improve typing predictions without accessing user data.
- Differential Privacy: By injecting statistical noise into datasets, differential privacy ensures individual data points cannot be traced. Apple uses this approach to gather usage insights while safeguarding user identities.
2. AI-Driven Data Anonymization
Data anonymization ensures that datasets can be used for analysis without compromising personal privacy. AI is making anonymization faster, more accurate, and adaptable to large-scale data.
Real-World Application:
- Healthcare providers can anonymize patient data using AI before sharing it with researchers. For example, an AI algorithm might mask identifiers like names and dates of birth while preserving patterns useful for medical studies.
- In the financial sector, AI-driven anonymization tools help organizations comply with regulations like GDPR by removing personally identifiable information (PII) from transaction records.
3. AI for Privacy Compliance Automation
As global data privacy laws evolve, ensuring compliance has become increasingly complex. AI-powered tools now assist organizations in navigating regulatory landscapes by automating privacy management tasks.
Notable Use Cases:
- Data Mapping: AI identifies where personal data resides across an organization’s systems, enabling better control and compliance.
- Consent Management: Platforms like OneTrust leverage AI to track user consent preferences, ensuring businesses meet obligations under laws such as CCPA and India’s DPDPA.
- Incident Reporting: AI can detect and report data breaches promptly, reducing the risk of non-compliance fines. For example, IBM’s AI-based security tools help organizations respond to threats within hours rather than days.
4. AI in Biometric Data Protection
Biometric authentication methods like facial recognition and fingerprint scanning are becoming mainstream, but they come with significant privacy concerns. AI is helping enhance the security of biometric data.
How It Works:
- On-Device Processing: Instead of sending biometric data to cloud servers, AI processes it locally on devices. Apple’s Face ID, for example, stores and processes facial data on the user’s iPhone, preventing external access.
- Anti-Spoofing Mechanisms: AI algorithms can detect spoofing attempts, such as fake fingerprints or photos, ensuring only genuine users are authenticated.
- Secure Templates: AI creates encrypted templates of biometric data, which cannot be reverse-engineered into the original format, reducing the risk of identity theft.
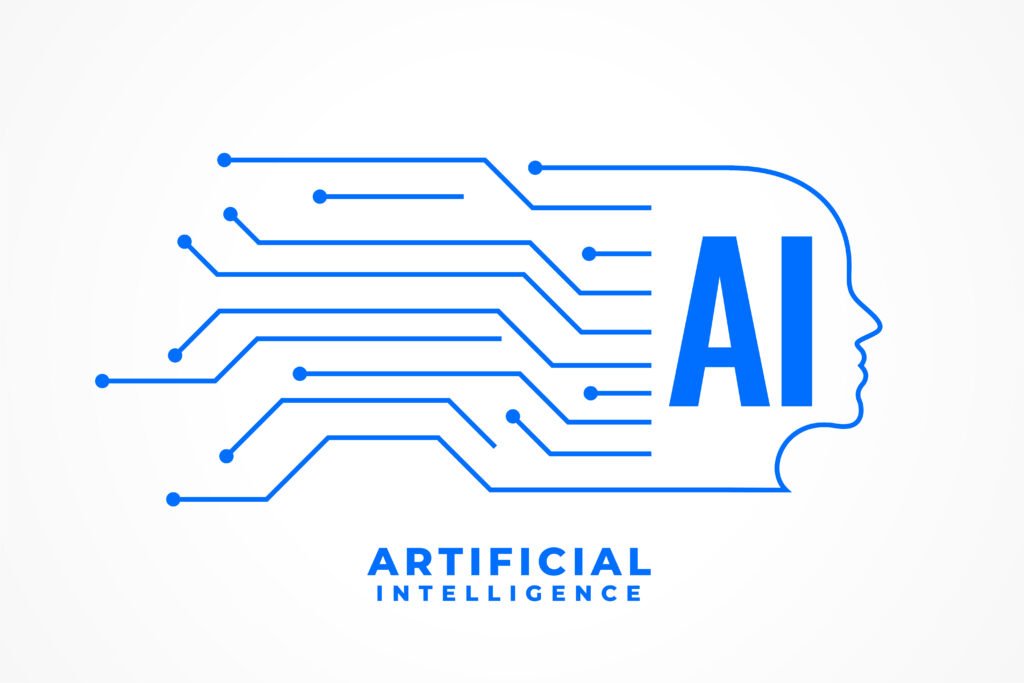
5. Ethical AI and Transparent Algorithms
As AI systems become more embedded in decision-making, ensuring they operate ethically and transparently is critical. Transparency builds trust and allows users to understand how their data is being used.
Emerging Practices:
- Explainable AI (XAI): XAI focuses on making AI decisions interpretable. For instance, financial institutions using AI for credit scoring are now required to explain the factors influencing an applicant’s score, ensuring fairness.
- Bias Mitigation: AI models often inherit biases from training data. Organizations are employing AI to identify and mitigate biases, particularly in hiring, lending, and policing systems. For example, LinkedIn’s AI ensures job recommendations are equally distributed across demographics.
- Regulatory Compliance: AI transparency aligns with upcoming regulations like the EU’s AI Act, which mandates disclosure of automated decision-making processes.
Challenges and Considerations
While these trends promise significant advancements, they also pose challenges:
- Balancing Innovation and Privacy: Organizations must innovate responsibly, ensuring that data privacy is never sacrificed for efficiency.
- Complex Implementation: Deploying AI-driven privacy solutions requires significant investment in technology and expertise.
- Global Variations in Privacy Laws: AI solutions must be adaptable to diverse regulatory environments, adding complexity to global operations.
Conclusion
In 2025, AI will play a dual role in advancing data privacy and addressing the challenges associated with it. From federated learning to ethical AI practices, these trends will shape the future of privacy-focused innovation. By embracing these advancements responsibly, organizations can build trust, comply with regulations, and unlock the full potential of AI without compromising user data.
Subscribe to our newsletter for the latest insights on AI, law, and technology. Join our community of IT and legal professionals to stay ahead of the curve!